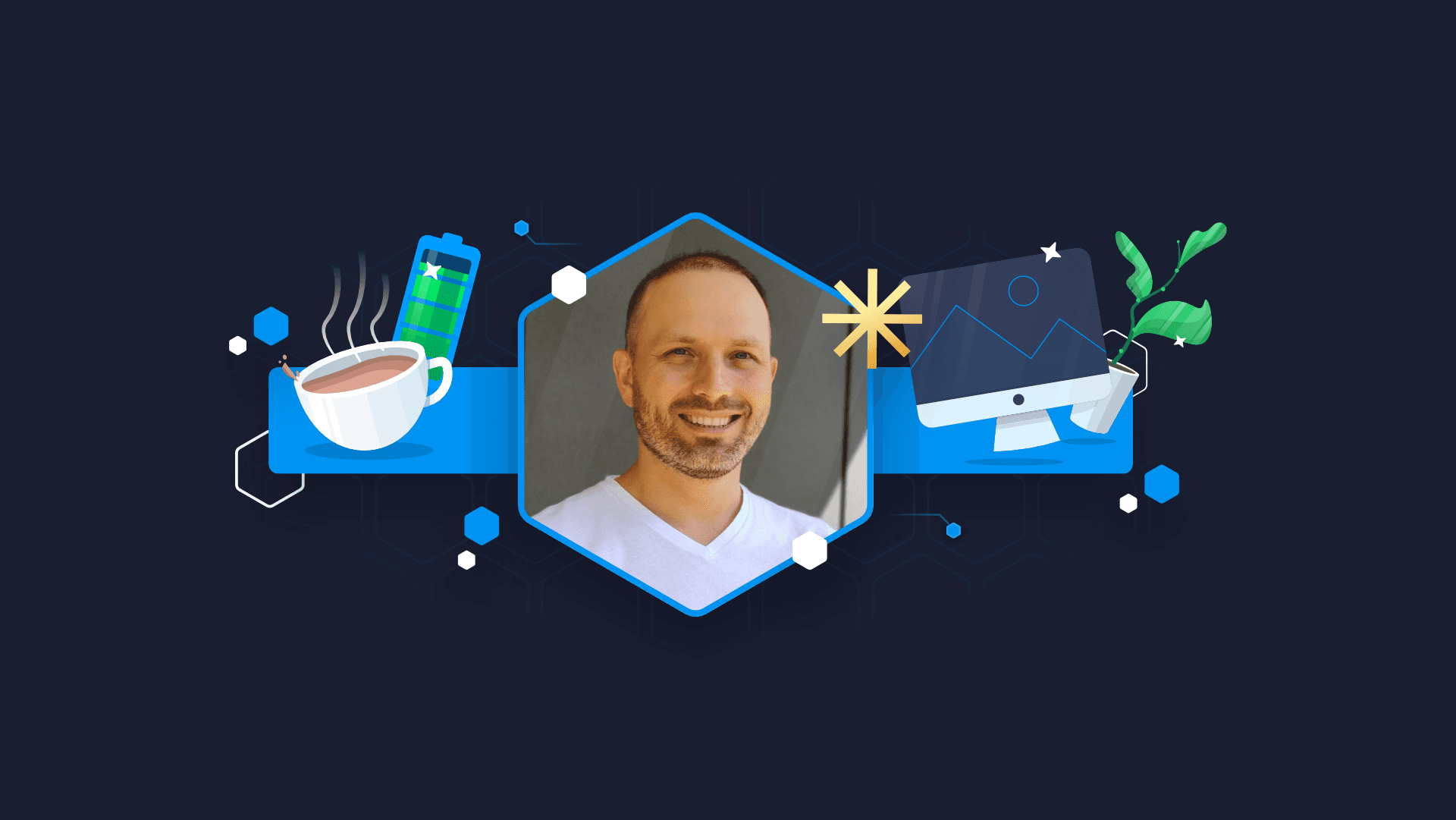
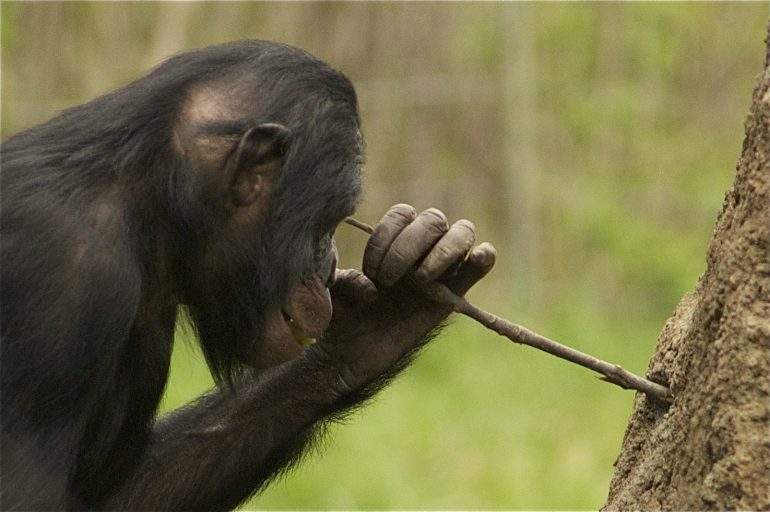
Data for Good: AI for Wildlife Image Classification to Analyze Camera Trap Datasets
Human activity has put enormous pressure on natural habitats across the world. Many species are finding it difficult to survive in the era of climate change and environmental degradation. Animals forced into smaller areas succumb to new diseases, which can spread to humans and cause pandemics. The question we ask ourselves is how can AI and ML be implemented to stop this. Is there potential here for wildlife image classification?
Biodiversity conservation practitioners and researchers are working to alleviate and reverse these processes through monitoring and protecting wild species. Increasingly they utilize cutting-edge technologies to support and streamline their work. Camera traps have become a ubiquitous tool for these purposes, and now machine learning offers further advancements by exponentially increasing the speed of wildlife image classification.
As part of our Data for Good initiative, we were given the opportunity to contribute to the work of biodiversity conservationists at the National Parks Agency in Gabon in collaboration with experts from the University of Stirling. We are proud to announce that we will use our computer vision skills in a project which recently received support from the Google for Education fund. Read on to learn more about AI-assisted biodiversity conservation and our project to protect wildlife in Gabon.
Camera traps – opportunities and challenges
Camera trap technology (automated photography of animals) is becoming more affordable and more accessible to biodiversity conservationists and is becoming the gold standard for monitoring many elusive or rare terrestrial mammals. Machine learning methods are revolutionizing the analysis of large camera trap datasets (which often contain millions of images) by automating the identification of animals in photographs.
Panthera camera trap in Lopé National Park, Gabon
Gabon in Central Africa is one of the last refuges for tropical forest mammals and is home to 80% of the world’s remaining critically endangered forest elephants, as well as gorillas, chimpanzees, pangolins, mandrills, and many others. Camera traps are used throughout its 13 national parks to monitor mammal populations and to inform land management in unprotected areas (e.g. ecological impact assessments of logging concessions or palm-oil plantations). Recent re-discoveries of spotted hyena and African lions in Gabon suggest there is significant potential for other discoveries.
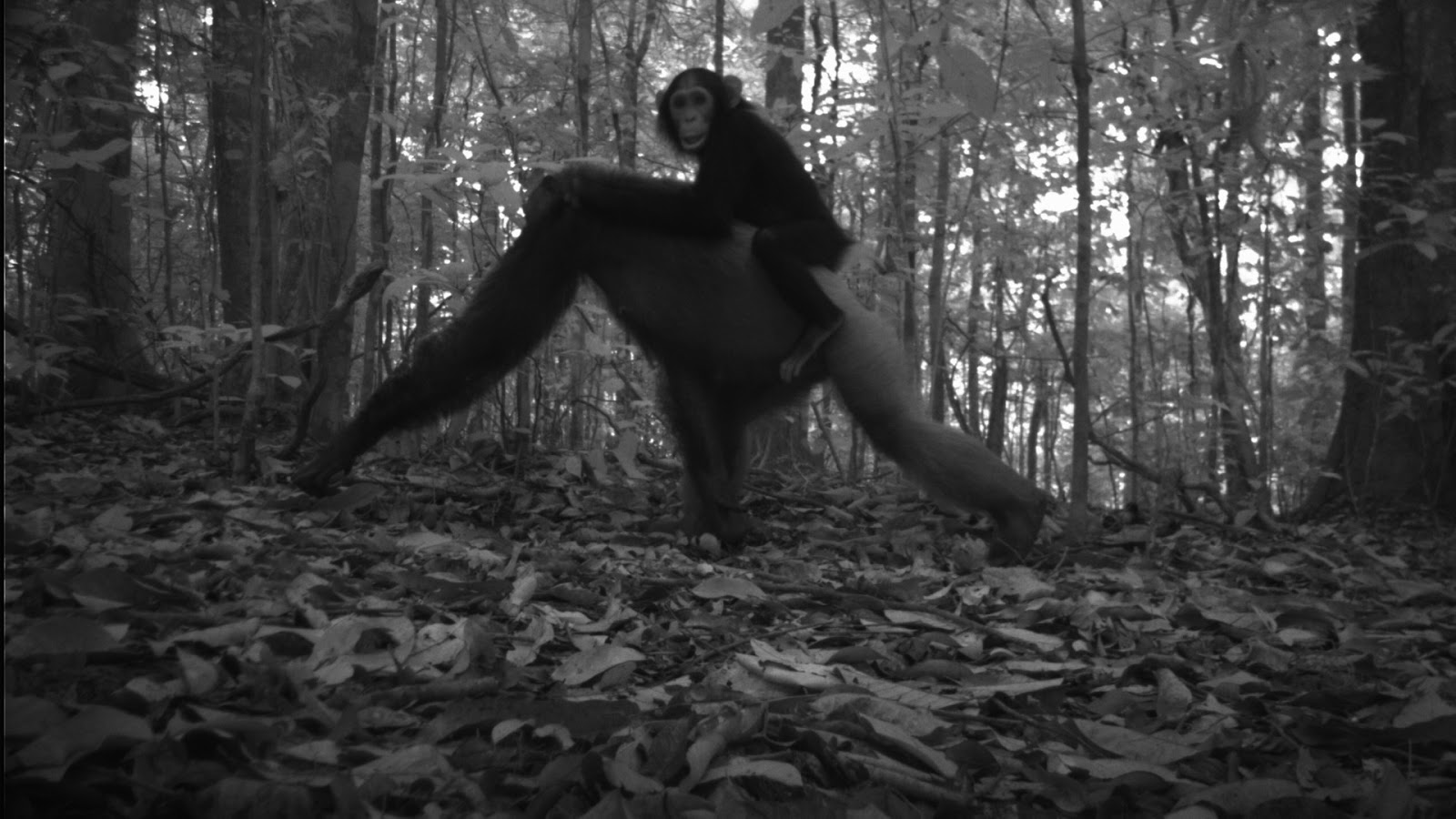
Chimpanzee mother and infant in Lopé National Park, Gabon
A recent large-scale biodiversity monitoring project in Gabon (a collaboration between the National Parks Agency of Gabon, Panthera Corp., and the University of Stirling) identified that land managers and conservationists are becoming increasingly frustrated with the speed and difficulty of analyzing camera trap data, despite significant technological advances. Delays can have a costly impact on endangered species such as elephants if changes in the distribution, abundance, or population structure (e.g. ratio of males to females) go unnoticed for months or even years. Cameras can also detect illegal activities (e.g. ivory poachers in protected areas), and it is imperative that these events are detected quickly to inform law enforcement.
Existing solutions and their limitations
The challenge of quickly classifying large image datasets has been described and addressed by academics and skilled practitioners alike. For instance, Norouzzadeh et al. (2018) discovered that deep learning techniques could automate animal identification for over 99% of images of wildlife in a dataset from the Serengeti Ecosystem in northern Tanzania. They showed that “automatically, accurately, and inexpensively collecting such data could help catalyze the transformation of many fields of ecology, wildlife biology, zoology, conservation biology, and animal behavior into ‘big data’ sciences.”
Tabak et al. (2018) provide further support for the utilization of machine learning for wildlife image classification in a resource-constrained context. First, they replicate the results from Norouzzadeh et al. (2018) showing 98% accuracy of an image classification model developed using a dataset from the United States. Second, the authors show that this same model could be successfully reused for out-of-sample datasets from Canada and Tanzania. This lends credibility to the idea of developing a universal model, which could be used globally. Third, they successfully run the model offline on a laptop computer.
This is a fast-developing area of research and there are other solutions that aim to tackle the issue of analyzing the vast amounts of data generated from camera traps. Whilst novel, they often do not take into consideration the limited resources available to conservationist practitioners, not only in the African context but also in other regions where biodiversity conservation is underfunded.
For instance, Wildlife Insights is a new cloud-based solution. It utilizes deep learning techniques for automatic wildlife image classification. However, it necessitates access to high-speed internet, proprietary data management systems, and other tools which are both costly and require specialized IT knowledge.
Solutions provided by scholars such as Tabak et al. (2018) can run offline on basic machines, however, they require significant expertise in the use of data science tools such as R and computer programming and they are not standalone applications. This precludes their use by experts from other domains.
Overall, existing tools typically require users to have some knowledge of computer programming and/or access to a fast internet connection (e.g. to use cloud-based platforms such as Zamba, Wildlife Insights, or the Marco Willi classifier). This poses a significant problem for many conservationists, who are only rarely experienced in computer programming and who often work in remote regions with little or no internet access. Furthermore, the current focus of machine-learning tools in Africa has been for savannah species, and these can be unsuitable for forest species. To allow conservationists to fully exploit the huge potential of camera traps for species monitoring, protection, and discovery, there is a need for simple software tools that can implement cutting-edge machine learning algorithms on basic hardware without internet access.
Appsilon’s approach to solving the problem
In building the Data for Good initiative at Appsilon we advocate an exploratory approach. My experiences working in international development led me to believe that many Western-developed, techno-centric solutions do not bring the anticipated results to end-users outside of this demographic, because they fail to appreciate the more complex reality of the context in which they are applied. Designers tend to look for a problem to solve with an exciting technological development rather than the other way around. To prevent repeating this mistake we engage with specialists in the respective fields, where machine learning and other cutting-edge technological solutions can provide assistance. To this end, we entered a collaboration with Dr. Robin Whytock and Dr. Katharine Abernethy, who are world-class experts on biodiversity conservation, and Robin is also an experienced data science practitioner.
Together we set out to integrate both new and existing machine learning algorithms into a simple-to-install and easy-to-use, an open-source software tool that can automatically analyze camera trap data without internet access. New algorithms will be created using a recently collated, unique database of 1.6 million labeled images taken across Central Africa during the last decade. The project will initially focus on Central African forest species in Gabon, but will also expand to encompass all of the Central African forest zones.
Since February 2018 Robin has worked for Gabon’s National Park Agency leading a large-scale biodiversity monitoring program (funded by the EU’s ECOFAC6 program) that uses 200 camera traps to survey mammal populations in Lopé and Waka National Parks (comprising 7000 km2 of continuous forest). The aim of this project has been to build capacity for long-term, scientifically rigorous biodiversity monitoring in both national parks and also within the National Parks Agency as a whole. Dr. Whytock will use the experience gained from working with local research teams in Gabon for the past two years to help us design and build a tool that is truly useful to local conservation managers, who have limited internet access and only basic computing skills, but who are increasingly using camera traps to monitor wildlife at larger spatial scales and for longer time periods.
Brice Momboua of the Lope-Waka ECOFAC6 biomonitoring project training ecoguards and research assistants about camera trapping methods in the Lope National Park, Gabon
Our plan
We will soon run an intense Sprint to create a pilot version of the solution, which will be available for Windows OS and meet the basic requirements of conservationists working in Gabon. We will finance this MVP using our own funds through our Data for Good initiative, and with Google Cloud Platform credits granted to Professor Abernethy by the Google for Education fund. We will use the credits to train our ML model.
In addition to this MVP, we would like to build a fully open-source multi-platform AI solution for wildlife image classification that will be available globally. If the MVP is successful, we plan to launch the large-scale project in the second half of this year.
Make sure to visit our blog in the coming weeks as we will be publishing updates about our progress. These updates will include a more technical discussion of the methods we are using to build the ML vision model and app, including the UX/UI design approach that should allow us to meet the needs of non-technical biodiversity researchers.
In the meantime, if you want to learn about image classification models in the context of wildlife preservation, check out our CTO Marek Rogala’s blog post about our recent participation in the Hakuna-ma Data competition organized by DrivenData, which asked participants to build an algorithm for wildlife detection that would generalize well across time and locations.
The content for this blog post has been developed with significant support from Dr. Robin Whytock.